Introduction
Welcome to Week 3. This week you will learn about some of the foundational principles for the statistical analysis of data. The principles of sample, population, variables, and levels of measurement play into the development of research studies as well as the critical evaluation of research studies.
Learning Outcomes
At the end of this lesson, you will be able to:
- Differentiate between a population and a sample.
- Discuss techniques for randomly sampling a population for a study.
- Define independent and dependent variables.
- Select a research article and evaluate the IV, DV, and level of measurement.
- Differentiate levels of measurement for statistical analysis.
Types of Sampling Methods
Review the following video to learn more about sampling:
Types of Sampling Methods TranscriptLinks to an external site.
Evidence-Based Pyramid—Levels of Evidence
View the following to learn about the levels of evidence and sources of each.
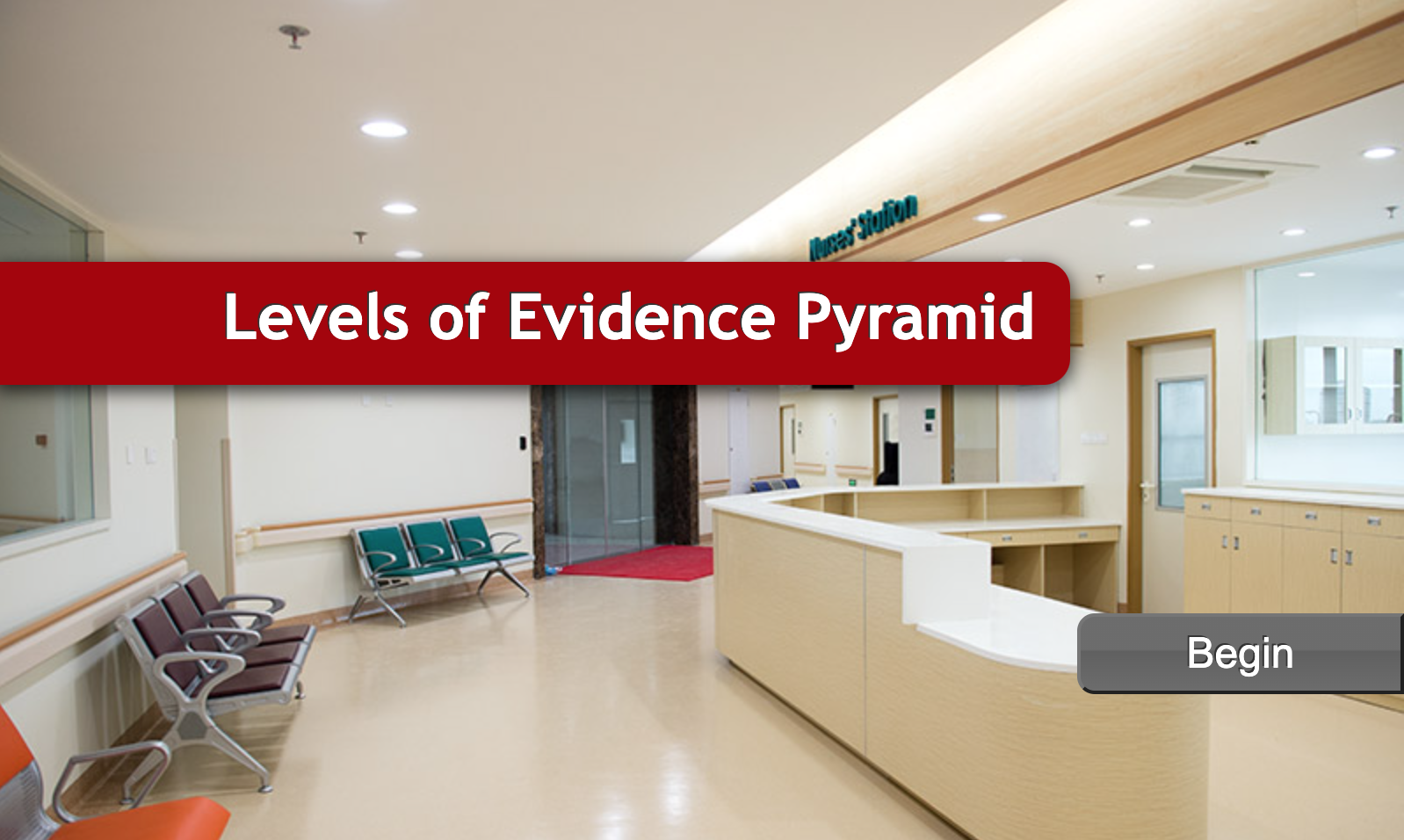
Levels of Evidence Pyramid TranscriptLinks to an external site.
The EBP pyramid may be used as a guideline for selecting the best evidence to answer a clinical foreground question. The types of studies higher up the pyramid typically offer increased relevance to the clinical setting. However, fewer studies may be available and you may not find the highest level of evidence to answer your clinical foreground question. In that case, move down the pyramid and explore other types of studies.
Click here to ORDER an A++ paper from our MASTERS and DOCTORATE WRITERS: NUR 705 Assignment 3.1: Levels of Measurement in Articles
Independent and Dependent Variables
Review the video on independent and dependent variables.
Independent and Dependent Variables TranscriptLinks to an external site.
Levels of Measurement
Review the video on Levels of Measurement:
Levels of Measurement TranscriptLinks to an external site.
Levels of Measurement Transcript
Quantitative researchers measure variables to answer their research question. The level of measurement that is used to measure a variable has a significant impact on the type of test researchers can do with their data, and therefore the conclusions they can come to. The higher the level of measurement, the more statistical tests that can be run with the data. That is why it is best to use the highest level of measurement possible when collecting information.
In this video, nominal, ordinal, interval and ratio levels will be described in order from the lowest to the highest level of measurement. By the end of this video you should be able to identify the level of measurement being used in this study. You will also be familiar with types of tests that can be done with each level.
To remember these levels of measurement in order, use the acronym, NOIR, or noir. The level of measurement of a variable depends on the nature of that variable as well as how the researcher collects the data. For example, some variables, like gender, can only be measured in a nominal way. Other variables, like household income, can be measured at multiple levels, depending on how the question is asked.
The nominal level of measurement is the lowest level. Variables in this study are placed into mutually exclusive categories. Each category has a criteria that a variable either has or does not have. There is no natural order to these categories. The categories maybe assigned numbers, but the numbers have no meaning because they are simply labels.
For example, if we categorize people by hair color, people with brown hair do not have more or less of a characteristic than those with blonde hair. Nominal sounds like name, so it is easy to remember that at a nominal level you are simply naming categories. Nominal data may be considered dichotomous or categorical. Dichotomous data falls into one of two categories, like male and female, or yes and no. Categorical data have more than two possible values, such as marital status or group membership. Sometimes researchers refer to nominal data as categorical or qualitative because it is not numerical.
Since nominal data is simply categorical it allows for the few statistical tests. It makes sense to report the number or percentage of people who are male or female in a particular group. This data is often presented in bar or pie charts. The only measure of central tendency that makes sense with nominal data is the mode. Many other statistical tests just do not make sense from nominal data.
For example, since there is no natural way to order nominal data you cannot find a median or middle number. Likewise, you cannot calculate a mean gender since numerical value for the data exists. Ordinal data is also considered categorical. The difference between nominal and ordinal data is that the categories have a natural order to them. You can remember that because ordinal sounds like order.
Numbers are assigned to categories but they are arbitrary. They are simply used to establish a ranking and there is no absolute zero. While there is an order, it is also unknown how much distance is between each category. The intervals between each number are therefore not necessarily equal.
Ordinal skills are often used to measure attitudes and perceptions. For example, a survey may ask how satisfied a customer is on a scale from very dissatisfied to very satisfied.
Nurses often use an ordinal scale to get patients to rank their pain on a scale from one to ten. This data is ordinal, since it is unknown whether the intervals between each value are equal. On a ten point scale, the difference between a nine and a ten is not necessarily perceived to be the same as the difference between a three and a four. All we know is that if the patient rates their pains as an eight now, and a four after receiving pain medication, the pain is decreased. We cannot accurately measure how much the pain has decreased, since we do not know the difference between the points on the scale. It would be inaccurate to claim that the patient was in twice as much paid before receiving the pain medication. Likewise, you cannot say that one patient is in twice as much pain as another using this scale.
Remember that the values in an ordinal scale simply express an order. All nominal level tests can be run on ordinal data. Since there is an order to the categories, the numbers assigned to each category can be compared in limited ways beyond nominal level tests. It is possible to say that members of one category have more of something than the members of a lower ranked category. However, you do not know how much more of that thing they have because the difference cannot be measured.
To determine central tendency, the categories can be placed in order and a median can now be calculated in addition to the mode. Since the distance between each category cannot be measured, the types of statistical tests that can be used on this data are still quite limited. For example, the mean or average of ordinal data cannot be calculated because the difference between the values on the scale is not known.
Interval level data is ordered like ordinal data, but the intervals between each value are known and equal. Therefore, the difference between two values is meaningful for interval variables. The zero point is arbitrary, since a score of zero does not actually mean that the variable does not exist. Zero simply represents an additional point of measurement.
For example, tests in school are interval level measurement of student knowledge. If you scored a zero on a math test, it does not mean you have no knowledge. Yet, the difference between the 79 and 80 on the test is measurable and equal to the difference between an 80 and an 81.
Temperature, if measured in degrees Fahrenheit or Celsius, is another good example of interval measurement. On the Fahrenheit scale, the difference between a temperature of 37 degrees and 38 degrees is the same as the difference between 89 degrees and 90 degrees. The zero is arbitrary, since the temperature of zero degrees does not mean that there is no temperature.
With interval level scales, there is a direct measurable quantity, In addition, zero does not represent the absolute lowest value. Instead, it is a point on the scale with numbers both above and below it. If you know that the word interval means space in between it makes remembering what makes this level of measurement different easy.
Interval scales not only tell us about order, but also about the value between items on a scale. Since the distance between points on the scale is measurable and equally split, it is possible to do more statistical test with the data. The mean, median and mode can all be calculated with interval data. The standard deviation can also now be calculated. However, the problem with performing statistical tests on interval scales is that they don’t have a true zero. Therefore, it is impossible to multiply, divide or calculate ratios.
Ratio measurement is the highest level possible for data. Like interval data, ratio data is ordered with known and measurable intervals between each value. What differentiates it from interval level data is that the zero is absolute. The zero occurs naturally and signifies the absence of the characteristic being measured. Remember, that ratio ends in an 0, therefore there is a zero.
Typically, this level of measurement is only possible with physical measurements, like height, weight and length. Any statistical test can be used with ratio level data as long as it fits with the study question end design. It is possible to compare amounts of the variable and make a claim that one is twice as much as the other. Remember, that when working with ratio variables, but not interval variables, you can look at the ratio of two measurements.
Remembering the basic differences can help you remember the levels of measurement. Nominal is named, ordinal is ordered, interval has a known interval or difference, ratio has a true zero.
To decide what level of measurement a particular variable is at, ask yourself these questions in order. First, is the variable ordered? If not, the variable is nominal. If it is ordered, ask yourself if there are equal distances between values. If not, the variable is ordinal. If values are equally spaced, ask yourself if a value of zero actually means that the variable being measured does not exit. If not, the variable is interval. If the zero means none, the variable is ratio because the zero is absolute.
The level of measurement dictates the appropriate statistical test that can be used. One of the reasons for learning about levels of measurement is so you know a statistical test can be performed on different types of data. That way you can avoid making mistakes in your own work and critique the work of others.
Be aware that some people gather ordinal data and treat it like interval data, once numbers are assigned to it. Researchers need to be careful not to make interval and ratio claims about ordinal data. Be careful not to claim that something is twice as much as something else if the data were not collected at the appropriate level.
Classifications of some forms of data are debated. For example, some researchers treat the measurement of intelligence as ordinal, while others treat it as interval. Likewise, money in the bank account may be considered ratio since having a balance of zero means you don’t have any money. However, others argue it is interval, since it is possible to have a negative balance, which makes the zero point simply another point of measurement. So, what level do you think it is? Can you think of any other controversial examples? Comment below to start a discussion.
What is important to know when reviewing an article is how the data was collected so you can identify if the appropriate statistical tests were used to analyze the data. If you are doing research, try to collect data in the highest form possible, so a wider variety of tests can be performed on it. Sometimes, how you ask the question will determine what level your data is at. Knowing the level of measurement for your data will help you avoid making mistakes, like taking the average of people’s marital status.
To help you remember what you need to know about the levels of measurement, try making a simple study table to include in your notes. It is helpful to include an example in the chart that will help you remember each level.
For more information, you can check out some of my related videos or website. You are also welcome to subscribe for regular updates. If there is something specific you are looking for, or would like created, please comment and let me know.
Thank you for watching.